Jun 1, 2025
What Will Fraud Detection Look Like in 2030?
What Will Fraud Detection Look Like in 2030?
What Will Fraud Detection Look Like in 2030?
Graph Neural Networks (GNNs) are poised to revolutionize how we combat the pervasive threat of evolving fraud tactics. Bureau predicts that by 2030, most organisations around the world will base their fraud detection methods on GNNs rather than rule-based systems. Here's why.
Mehul Dhikonia



Table of Contents
Financial fraud is a global epidemic, costing the world trillions of dollars annually. In 2023 alone, consumers lost a staggering $5.8 billion to fraud in the U.S., while businesses faced an even higher toll. This relentless onslaught of fraudulent activity is increasingly sophisticated, exploiting vulnerabilities in traditional detection systems and eroding trust in the digital economy.
The need for innovative solutions is urgent, and Graph Neural Networks (GNNs) are poised to revolutionize how we combat this pervasive threat. Bureau is making the prediction that by 2030, most organisations around the world will base their fraud detection methods on GNNs and not rule based systems.
The limitations of legacy fraud detection systems
Legacy fraud detection relies on rule-based engines. These act like digital detectives, pre-programmed with conditions that flag suspicious activity. Imagine a set of instructions saying "block transactions over $1,000 from a new device." If a transaction meets this rule, it gets flagged for review.
These systems, while straightforward, are inherently reactive. They rely on predefined rules and thresholds that quickly become outdated as fraudsters evolve their tactics. The result is a high rate of false positives, frustrating customers, and straining resources.
The toll of inadequate fraud prevention:
Financial Losses: Businesses and individuals alike face substantial financial losses due to fraud, impacting bottom lines and personal finances.
Eroding Trust: Fraud erodes trust in digital platforms and financial institutions, hindering economic growth and innovation.
Operational Costs: Investigating and remediating fraud cases consumes valuable time and resources.
Here is a comprehensive set of limitations that rule-based engines face.
Graph Neural Networks: New ways of looking at fraud detection
GNNs offer a fundamentally different approach to fraud detection. By modeling financial ecosystems as graphs, they can uncover hidden connections, detect subtle patterns, and adapt to evolving fraud tactics. This makes GNNs particularly effective in addressing the shortcomings of legacy systems.
Key advantages of GNNs:
Relationship-Centric Analysis: GNNs excel at understanding the complex relationships between entities like customers, transactions, devices, and accounts, revealing hidden links that traditional models might miss.
Adaptability: GNNs can learn and evolve continuously, staying ahead of emerging fraud patterns and minimizing false positives.
Contextual Awareness: GNNs consider the broader context of each transaction, incorporating information about the customer's profile, device history, and network connections. This holistic view enables more accurate risk based assessments.
How GNNs work in fraud detection
A graph neural network (GNN) is a type of artificial intelligence specifically designed to analyze data structured as graphs. These graphs consist of nodes (data points) connected by edges (relationships).
Here are the core components of a GNN:
Node embeddings: GNNs learn to represent each node in the graph as a numerical vector, encoding its features and the information from its surrounding neighborhood.
Message passing: GNNs propagate information between nodes through the edges of the graph. This message-passing mechanism allows the model to capture the complex dependencies and relationships within the financial ecosystem.
Aggregation: At each node, the received messages are aggregated to update the node's representation, incorporating information from its neighbors.
Prediction: The final node representations can be used for various tasks, such as predicting fraudulent transactions, identifying high-risk accounts, or detecting money laundering schemes.

The future of fraud prevention: A multi-layered defense
The most effective fraud prevention strategies combine the strengths of multiple technologies:
GNNs: Provide a powerful foundation for understanding relationships and uncovering hidden patterns.
AI-Powered Anomaly Detection: Identify unusual behavior and outliers that may indicate fraudulent activity.
Behavioral Biometrics: Analyze user interactions to detect anomalies in typing patterns, mouse movements, and other behavioral signals. See how we leverage this for frictionless authentication.
Real-Time Data Analytics: Monitor transaction streams in real-time to identify emerging threats and anomalies.
By adopting a multi-layered approach that combines these cutting-edge technologies with established methods, organizations can create a more resilient and secure financial ecosystem for everyone.
Companies like Bureau are pioneers in harnessing the power of GNNs and AI to build robust fraud detection solutions. Their comprehensive suite of tools, including money mule detection, device risk assessment, and identity network analysis, empowers businesses to proactively protect themselves against fraud.
Financial fraud is a global epidemic, costing the world trillions of dollars annually. In 2023 alone, consumers lost a staggering $5.8 billion to fraud in the U.S., while businesses faced an even higher toll. This relentless onslaught of fraudulent activity is increasingly sophisticated, exploiting vulnerabilities in traditional detection systems and eroding trust in the digital economy.
The need for innovative solutions is urgent, and Graph Neural Networks (GNNs) are poised to revolutionize how we combat this pervasive threat. Bureau is making the prediction that by 2030, most organisations around the world will base their fraud detection methods on GNNs and not rule based systems.
The limitations of legacy fraud detection systems
Legacy fraud detection relies on rule-based engines. These act like digital detectives, pre-programmed with conditions that flag suspicious activity. Imagine a set of instructions saying "block transactions over $1,000 from a new device." If a transaction meets this rule, it gets flagged for review.
These systems, while straightforward, are inherently reactive. They rely on predefined rules and thresholds that quickly become outdated as fraudsters evolve their tactics. The result is a high rate of false positives, frustrating customers, and straining resources.
The toll of inadequate fraud prevention:
Financial Losses: Businesses and individuals alike face substantial financial losses due to fraud, impacting bottom lines and personal finances.
Eroding Trust: Fraud erodes trust in digital platforms and financial institutions, hindering economic growth and innovation.
Operational Costs: Investigating and remediating fraud cases consumes valuable time and resources.
Here is a comprehensive set of limitations that rule-based engines face.
Graph Neural Networks: New ways of looking at fraud detection
GNNs offer a fundamentally different approach to fraud detection. By modeling financial ecosystems as graphs, they can uncover hidden connections, detect subtle patterns, and adapt to evolving fraud tactics. This makes GNNs particularly effective in addressing the shortcomings of legacy systems.
Key advantages of GNNs:
Relationship-Centric Analysis: GNNs excel at understanding the complex relationships between entities like customers, transactions, devices, and accounts, revealing hidden links that traditional models might miss.
Adaptability: GNNs can learn and evolve continuously, staying ahead of emerging fraud patterns and minimizing false positives.
Contextual Awareness: GNNs consider the broader context of each transaction, incorporating information about the customer's profile, device history, and network connections. This holistic view enables more accurate risk based assessments.
How GNNs work in fraud detection
A graph neural network (GNN) is a type of artificial intelligence specifically designed to analyze data structured as graphs. These graphs consist of nodes (data points) connected by edges (relationships).
Here are the core components of a GNN:
Node embeddings: GNNs learn to represent each node in the graph as a numerical vector, encoding its features and the information from its surrounding neighborhood.
Message passing: GNNs propagate information between nodes through the edges of the graph. This message-passing mechanism allows the model to capture the complex dependencies and relationships within the financial ecosystem.
Aggregation: At each node, the received messages are aggregated to update the node's representation, incorporating information from its neighbors.
Prediction: The final node representations can be used for various tasks, such as predicting fraudulent transactions, identifying high-risk accounts, or detecting money laundering schemes.

The future of fraud prevention: A multi-layered defense
The most effective fraud prevention strategies combine the strengths of multiple technologies:
GNNs: Provide a powerful foundation for understanding relationships and uncovering hidden patterns.
AI-Powered Anomaly Detection: Identify unusual behavior and outliers that may indicate fraudulent activity.
Behavioral Biometrics: Analyze user interactions to detect anomalies in typing patterns, mouse movements, and other behavioral signals. See how we leverage this for frictionless authentication.
Real-Time Data Analytics: Monitor transaction streams in real-time to identify emerging threats and anomalies.
By adopting a multi-layered approach that combines these cutting-edge technologies with established methods, organizations can create a more resilient and secure financial ecosystem for everyone.
Companies like Bureau are pioneers in harnessing the power of GNNs and AI to build robust fraud detection solutions. Their comprehensive suite of tools, including money mule detection, device risk assessment, and identity network analysis, empowers businesses to proactively protect themselves against fraud.

Solutions
Resources
Solutions
Solutions
Industries
Industries
Resources
Resources
Company
Company
Solutions
Solutions
Industries
Industries
Resources
Resources
Company
Company
© 2025 Bureau . All rights reserved. Privacy Policy. Terms of Service.
© 2025 Bureau . All rights reserved.
Privacy Policy. Terms of Service.
Follow Us
Leave behind fragmented tools. Stop fraud rings, cut false declines, and deliver secure digital journeys at scale
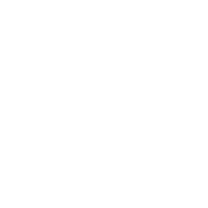
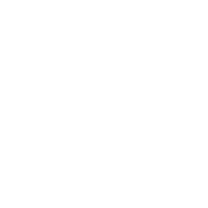

Contact Bureau

Contact Bureau
Leave behind fragmented tools. Stop fraud rings, cut false declines, and deliver secure digital journeys at scale
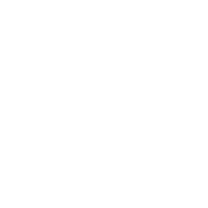
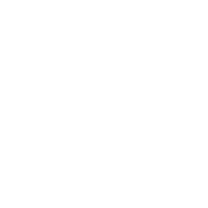

Contact Bureau

Contact Bureau