Jun 1, 2025
The Definitive Guide to Fraud Detection and Prevention
The Definitive Guide to Fraud Detection and Prevention
The Definitive Guide to Fraud Detection and Prevention
In this Fraud Detection and Prevention guide, we talk about different types of fraud across industries, common challenges, the use of fraud analytics, and much more!
Bureau Team



Table of Contents
Fraud detection refers to identifying suspicious activities in digital transactions. It could be spotting instances of unauthorized access, a serious case of identity theft in a corporate environment, or even payment fraud in everyday B2C transactions.
In the past, fraud detection was done manually with the help of tracking audit trails, paper documents, and so on. Today, we have at our disposal, AI, machine learning, and several rule-based systems to flag potential frauds in real time.
Fraud detection, to be effective, must work proactively - by blocking fraudsters and fraudulent attempts in real-time before they cause impact. This is done by combining various fraud prevention solutions like risk assessment, user authentication, and behavioral analytics to stop cybercriminals from exploiting financial systems.
Types of Fraud
The spread of modern-day fraud is not limited to a specific region but is wreaking havoc on personal lives and corporate stability. It is necessary that you know of all the possible types of fraud across different industries and be prepared to fight them.
Some of the common types of fraud are:
Payment Fraud
Identity Fraud
Banking & Financial Fraud
Credential-Based Fraud
Account & Transaction Manipulation Fraud
Social Engineering & AI-Powered Fraud
Mobile & App-Based Fraud
Payment Fraud
Payment frauds are the most common types of fraud used by cybercriminals. It is committed by manipulating online payment systems to make unauthorized transactions, inappropriate funds, or even exploit loopholes in refund policies.
There are three major subtypes of payment fraud:
Card-Not-Present (CNP) Fraud, where stolen card details are used for online purchases. This is possible in instances where physical card verification is not required, which is most of the online shopping transactions.
Chargeback Fraud is where the buyer disagrees to pay a legitimate charge to get a refund while refusing to return the product.
Account Takeover (ATO), wherein unauthorized access is gained to a user’s bank accounts or digital wallets and fraudulent transactions are carried out.
Identity Fraud
Identity fraud involves a fraudster impersonates a person but there are other ulterior motives as well. Identity fraud is typically done using stolen or fabricated personal documents. We are seeing reports of deepfakes, powered by AI, being used by fraud for identity fraud.
Credential-Based Fraud
This type of fraud occurs when attackers use stolen or guessed login credentials to gain access to user accounts and conduct financial crimes. Many cybercriminals leverage data breaches, phishing, or automated attacks to obtain credentials and then, use Credential Stuffing to gain unauthorized access to your customer accounts.
Social Engineering & AI-Powered Fraud
Artificial Intelligence has led to a new breed of frauds that are difficult to track and even complicated to fight against. Social Engineering refers to cybercriminals using personal information gathered from a data breach or public sources (social media handles, for example) to convince a victim into surrendering sensitive information like OTP, CVV, etc. We have also seen the use of AI to mimic human behavior and even use AI-driven deception to mislead individuals.
Mobile App-Based Fraud
Mobile apps simplify digital banking and mobile payments. However, they also open a pandora's box of vulnerabilities to steal credentials, hijack accounts, and spread malware.
Some of the most common mobile app-based frauds include:
SIM Swap Fraud – Attackers take control of a victim’s phone number to bypass authentication.
Malware & Banking Trojans – Malicious and virus-infected apps that steal banking credentials or manipulate transactions.
Fake App Fraud – Fraudsters create counterfeit apps that mimic legitimate financial services to steal user data.
What Makes Fraud Detection Challenging?
Fraud detection is a never-ending race between cybercriminals and security teams. While companies invest in advanced fraud detection systems, fraudsters continuously adapt, manage to stay ahead, and find innovative ways to bypass new defenses.
Here are some of the biggest challenges businesses face in detecting and preventing fraud.
Reactive Fraud Detection Measures
Most fraud detection systems are reactive; that is, they act after an attack has already occurred. Organizations analyze past fraud patterns, then implement rules and models to prevent future incidents. But fraudsters evolve faster, always staying one step ahead. Also, fraudsters rarely deploy the same tactic again, making the reactive measures defunct. In other words, the reactive fraud detection measure may not detect the new fraud methodology for a long time until it breaches a dangerous threshold.
This is why, at Bureau, we have built a product that power your fraud detection systems with real time intelligence to actively fight fraud and block fraudsters.
High False Positives Lead to Poor Customer Experience
A fraud detection system must strike a balance between catching fraud and allowing legitimate transactions. Quite often, businesses set up complicated workflows in an attempt to ward off fraud. However, it could create false positives and also make things difficult for customers to perform even simple transactions. They could be frustrating for customers and lead to poor customer experience.
Fraud Detection Systems Require Continuous Tuning
We discussed earlier that cybercriminals are ahead of defense systems. This mandates the need for rules-based fraud detection systems to be constantly updated. Also, the use of AI and Machine learning models require a constant supply of fresh data to revise the rules. Without this, systems become obsolete and ineffective against new fraud schemes. Further, frauds are no longer carried out by individuals. They are done at scale with the help of AI bots. Automate phishing, credential stuffing, and synthetic identity are all carried out at a staggering rate by bots, which makes detection and prevention difficult.
This is where Bureau's risk intelligence will always be better against company's personal model. We work with leading companies across industries which, in turn, helps us ensure depth and accuracy in the intelligence we provide.
If you are curious about our capabilities and want to know more, fill this form and someone from our team will reach out.
Lack of Cross-Industry Collaboration
One of the harsh truths of the any industry is that institutions rarely share fraud intelligence. Even if they do, it is merely for compliance and not with the common objective of raising a common shield against cyber frauds. Also, there is the element of credibility and loss of public trust involved, which demotivates financial institutions from sharing fraud intel.
This gives fraudsters an opening to repeat frauds that have worked in one type of company. This loophole, combined with a reactive fraud detection model, works in their favor while creating adverse effects for the business and the concerned individuals.
Fraud Detection is Expensive and Resource-Intensive
Let’s face it. Fraud detection at scale is not something small and medium businesses can consider, mainly because of the cost involved.
According to PwC’s Economic Crime Survey 2024, 59% of C-suite executives of Billion-dollar firms agree that export controls have grown more complex, and more than half believe controls are being enforced more robustly than two years ago.
Further, to put up a defense against the latest crop of AI-driven frauds, businesses need to invest in an effective fraud detection system. This requires a combination of AI models, data analytics, and human fraud analysts, all of which cost an exorbitant amount of money, materials, and resources. This cost is a deterrent for many businesses.
The True Cost Of Fraud
According to Nasdaq’s Global Financial Crime Report, fraud scams and bank fraud schemes resulted in $485.6 billion in projected losses globally.
The situation is particularly concerning in the Asia-Pacific (APAC) region, where fraud incidents continue to rise. A report reveals that 58% of APAC organizations have experienced an increase in fraud year-over-year.
Additionally, for every dollar lost to fraud in the Asia Pacific, firms incur an actual cost of SGD 3.95, considering direct financial losses, recovery expenses, and operational disruptions.
The true cost of fraud is from three different angles.
Financial loss, the obvious result of fraud
Reputation damage is intangible, yet it leads to the loss of new and existing business
Regulatory risks, compliance nightmares, fines, penalties, restructuring, or even total shutdown of operations

Here is a detailed take on each of the angles.
Financial Loss
The immediate and direct impact of any type of fraud is the financial loss resulting from Account Takeover, unauthorized transactions, chargebacks, etc.
Every $100 lost to fraud causes businesses atleast $200 in losses. Beyond the face value of fraudulent transactions, businesses have to spend additional resources for internal investigations, legal proceedings, and inflated insurance costs in the years following the fraud incident.
Reputation Damage
Frauds invariably tarnish a company's reputation. It leads to erosion of customers' trust and subsequent churn of major accounts to competition.
If you, a consumer, hear about a famous bank facing a data breach or a customer from another famous brand defrauded, what will be your first reaction? The human psychology works in a way that a negative connotation with company's name sticks for longer as compared to a positive experience.
Regulatory Risks
Fraud incidents kick into action a long list of global compliances and risk mitigation measures. A fraud incident can kick off several investigations and resultant fines, sanctions, and increased scrutiny from regulatory bodies.
For instance, the Australian Securities & Investments Commission (ASIC) recently pursued legal action against HSBC for failing to protect its customers from scams, highlighting the regulatory risks associated with inadequate fraud prevention measures.
Tackling Fraud Globally, One Transaction at a Time
Fraud prevention in the digital world requires a proactive, multi-layered approach. A reactive approach, as we have already seen, is detrimental and even obsolete.
Businesses should double down on real-time fraud detection and AI-driven fraud monitoring and establish constantly evolving security protocols.
Based on the challenges discussed earlier—such as false positives, regulatory risks, and evolving fraud techniques—here’s how you can strengthen your fraud prevention efforts.
Implement AI-driven fraud detection to detect fraud patterns in real time, reducing false positives and improving accuracy.
Strengthen Identity Verification with Multi-Factor Authentication (MFA) like OTPs, biometrics, or security keys to prevent unauthorized account access.
Use Behavioral Biometrics. Tracking user behavior, such as real-time location and device ID, helps detect fraudulent activities.
Deploying Device Fingerprinting prevents fraudsters from using multiple stolen identities on the same device that go unnoticed with the use of Device ID.
Ensure Compliance with Regulatory Standards like PCI DSS, PSD2, and GDPR to ensure secure transactions while minimizing regulatory risks.
Raising user awareness about phishing scams, account takeovers, and fraud prevention best practices can help reduce vulnerability.
How Fraud Analytics Powers Proactive Fraud Detection and Prevention
According to the ACFE’s Occupational Fraud: A Report to the Nations, organizations utilizing proactive data analytics to combat fraud experience a 47% lower fraud loss compared to those without data analytics.
Fraud analytics can help in detecting, preventing, and mitigating fraud with data-driven insights. It helps analyze transaction patterns, user behavior, and historical fraud data, businesses can proactively identify risks and enhance their fraud prevention strategies.
Quantifying Losses and Measuring Fraud Impact
Fraud analytics helps businesses assess the financial impact of fraud. It helps place a finger on the actual cause and effect of fraud while acting as the base to determine the cost of fraud. The same data can also be used to calculate intangible losses that go beyond direct monetary losses.
Identifying Patterns and Fraud Techniques
By analyzing large datasets, fraud analytics detects recurring fraud patterns, common fraud MOs (Modus Operandi), and emerging threats, helping businesses stay ahead of fraudsters.
Pinpointing High-Risk Regions, Devices, and Account Types
Most digital frauds originate from specific regions or are carried out using specific devices. There are also account types that can be associated with certain frauds.
Fraud analytics enables businesses to geo-map fraudulent activities, identify suspicious device fingerprints, and flag risky accounts based on behavioral anomalies.
For example, a digital wallet provider, like Google Pay, detects that a specific city has a surge in account takeovers, prompting targeted security measures for users in that region.
Reducing False Positives and Optimizing Customer Experience
Fraud analytics helps businesses fine-tune fraud detection rules and ensure that their system is not flagging too many false positives. This reduces unnecessary transaction declines and ensures a frictionless customer experience.
Identifying Patterns and Fraud Techniques
The obvious benefit of fraud analytics is that it enables the analysis of large datasets. The analysis can unearth recurring fraud patterns, common fraud MOs (Modus Operandi), and emerging threat models that can help take proactive threat mitigation measures.
Fraud analytics is no longer optional - it's a necessity. By leveraging data-driven insights, businesses can proactively prevent fraud, minimize losses, and strengthen trust with customers.
Frequently Asked Questions (FAQ)
What is fraud detection, and why is it important?
Fraud detection is identifying and preventing fraudulent activities in digital transactions, new account opening, account logins, and operations. Businesses must prevent financial losses, protect customer data, and maintain regulatory compliance.
What are the biggest challenges in fraud detection?
Fraudsters constantly evolve their tactics, making detection difficult.
Balancing fraud prevention with a smooth customer experience.
High false positives can lead to unnecessary transaction declines.
Regulatory compliance requirements add complexity to fraud management.
How should businesses ensure compliance with fraud prevention regulations?
Businesses must follow and keep track of changes around regulations such as PCI DSS (for payment security), PSD2 (for strong customer authentication in Europe), and AML/KYC requirements (for financial institutions). For instance, SAMA's Counter-Fraud Framework mentions the use of authentication measures invloving Behavior and Device for fraud prevention. Fraud analytics helps in generating compliance reports and tracking suspicious activities.
Fraud detection refers to identifying suspicious activities in digital transactions. It could be spotting instances of unauthorized access, a serious case of identity theft in a corporate environment, or even payment fraud in everyday B2C transactions.
In the past, fraud detection was done manually with the help of tracking audit trails, paper documents, and so on. Today, we have at our disposal, AI, machine learning, and several rule-based systems to flag potential frauds in real time.
Fraud detection, to be effective, must work proactively - by blocking fraudsters and fraudulent attempts in real-time before they cause impact. This is done by combining various fraud prevention solutions like risk assessment, user authentication, and behavioral analytics to stop cybercriminals from exploiting financial systems.
Types of Fraud
The spread of modern-day fraud is not limited to a specific region but is wreaking havoc on personal lives and corporate stability. It is necessary that you know of all the possible types of fraud across different industries and be prepared to fight them.
Some of the common types of fraud are:
Payment Fraud
Identity Fraud
Banking & Financial Fraud
Credential-Based Fraud
Account & Transaction Manipulation Fraud
Social Engineering & AI-Powered Fraud
Mobile & App-Based Fraud
Payment Fraud
Payment frauds are the most common types of fraud used by cybercriminals. It is committed by manipulating online payment systems to make unauthorized transactions, inappropriate funds, or even exploit loopholes in refund policies.
There are three major subtypes of payment fraud:
Card-Not-Present (CNP) Fraud, where stolen card details are used for online purchases. This is possible in instances where physical card verification is not required, which is most of the online shopping transactions.
Chargeback Fraud is where the buyer disagrees to pay a legitimate charge to get a refund while refusing to return the product.
Account Takeover (ATO), wherein unauthorized access is gained to a user’s bank accounts or digital wallets and fraudulent transactions are carried out.
Identity Fraud
Identity fraud involves a fraudster impersonates a person but there are other ulterior motives as well. Identity fraud is typically done using stolen or fabricated personal documents. We are seeing reports of deepfakes, powered by AI, being used by fraud for identity fraud.
Credential-Based Fraud
This type of fraud occurs when attackers use stolen or guessed login credentials to gain access to user accounts and conduct financial crimes. Many cybercriminals leverage data breaches, phishing, or automated attacks to obtain credentials and then, use Credential Stuffing to gain unauthorized access to your customer accounts.
Social Engineering & AI-Powered Fraud
Artificial Intelligence has led to a new breed of frauds that are difficult to track and even complicated to fight against. Social Engineering refers to cybercriminals using personal information gathered from a data breach or public sources (social media handles, for example) to convince a victim into surrendering sensitive information like OTP, CVV, etc. We have also seen the use of AI to mimic human behavior and even use AI-driven deception to mislead individuals.
Mobile App-Based Fraud
Mobile apps simplify digital banking and mobile payments. However, they also open a pandora's box of vulnerabilities to steal credentials, hijack accounts, and spread malware.
Some of the most common mobile app-based frauds include:
SIM Swap Fraud – Attackers take control of a victim’s phone number to bypass authentication.
Malware & Banking Trojans – Malicious and virus-infected apps that steal banking credentials or manipulate transactions.
Fake App Fraud – Fraudsters create counterfeit apps that mimic legitimate financial services to steal user data.
What Makes Fraud Detection Challenging?
Fraud detection is a never-ending race between cybercriminals and security teams. While companies invest in advanced fraud detection systems, fraudsters continuously adapt, manage to stay ahead, and find innovative ways to bypass new defenses.
Here are some of the biggest challenges businesses face in detecting and preventing fraud.
Reactive Fraud Detection Measures
Most fraud detection systems are reactive; that is, they act after an attack has already occurred. Organizations analyze past fraud patterns, then implement rules and models to prevent future incidents. But fraudsters evolve faster, always staying one step ahead. Also, fraudsters rarely deploy the same tactic again, making the reactive measures defunct. In other words, the reactive fraud detection measure may not detect the new fraud methodology for a long time until it breaches a dangerous threshold.
This is why, at Bureau, we have built a product that power your fraud detection systems with real time intelligence to actively fight fraud and block fraudsters.
High False Positives Lead to Poor Customer Experience
A fraud detection system must strike a balance between catching fraud and allowing legitimate transactions. Quite often, businesses set up complicated workflows in an attempt to ward off fraud. However, it could create false positives and also make things difficult for customers to perform even simple transactions. They could be frustrating for customers and lead to poor customer experience.
Fraud Detection Systems Require Continuous Tuning
We discussed earlier that cybercriminals are ahead of defense systems. This mandates the need for rules-based fraud detection systems to be constantly updated. Also, the use of AI and Machine learning models require a constant supply of fresh data to revise the rules. Without this, systems become obsolete and ineffective against new fraud schemes. Further, frauds are no longer carried out by individuals. They are done at scale with the help of AI bots. Automate phishing, credential stuffing, and synthetic identity are all carried out at a staggering rate by bots, which makes detection and prevention difficult.
This is where Bureau's risk intelligence will always be better against company's personal model. We work with leading companies across industries which, in turn, helps us ensure depth and accuracy in the intelligence we provide.
If you are curious about our capabilities and want to know more, fill this form and someone from our team will reach out.
Lack of Cross-Industry Collaboration
One of the harsh truths of the any industry is that institutions rarely share fraud intelligence. Even if they do, it is merely for compliance and not with the common objective of raising a common shield against cyber frauds. Also, there is the element of credibility and loss of public trust involved, which demotivates financial institutions from sharing fraud intel.
This gives fraudsters an opening to repeat frauds that have worked in one type of company. This loophole, combined with a reactive fraud detection model, works in their favor while creating adverse effects for the business and the concerned individuals.
Fraud Detection is Expensive and Resource-Intensive
Let’s face it. Fraud detection at scale is not something small and medium businesses can consider, mainly because of the cost involved.
According to PwC’s Economic Crime Survey 2024, 59% of C-suite executives of Billion-dollar firms agree that export controls have grown more complex, and more than half believe controls are being enforced more robustly than two years ago.
Further, to put up a defense against the latest crop of AI-driven frauds, businesses need to invest in an effective fraud detection system. This requires a combination of AI models, data analytics, and human fraud analysts, all of which cost an exorbitant amount of money, materials, and resources. This cost is a deterrent for many businesses.
The True Cost Of Fraud
According to Nasdaq’s Global Financial Crime Report, fraud scams and bank fraud schemes resulted in $485.6 billion in projected losses globally.
The situation is particularly concerning in the Asia-Pacific (APAC) region, where fraud incidents continue to rise. A report reveals that 58% of APAC organizations have experienced an increase in fraud year-over-year.
Additionally, for every dollar lost to fraud in the Asia Pacific, firms incur an actual cost of SGD 3.95, considering direct financial losses, recovery expenses, and operational disruptions.
The true cost of fraud is from three different angles.
Financial loss, the obvious result of fraud
Reputation damage is intangible, yet it leads to the loss of new and existing business
Regulatory risks, compliance nightmares, fines, penalties, restructuring, or even total shutdown of operations

Here is a detailed take on each of the angles.
Financial Loss
The immediate and direct impact of any type of fraud is the financial loss resulting from Account Takeover, unauthorized transactions, chargebacks, etc.
Every $100 lost to fraud causes businesses atleast $200 in losses. Beyond the face value of fraudulent transactions, businesses have to spend additional resources for internal investigations, legal proceedings, and inflated insurance costs in the years following the fraud incident.
Reputation Damage
Frauds invariably tarnish a company's reputation. It leads to erosion of customers' trust and subsequent churn of major accounts to competition.
If you, a consumer, hear about a famous bank facing a data breach or a customer from another famous brand defrauded, what will be your first reaction? The human psychology works in a way that a negative connotation with company's name sticks for longer as compared to a positive experience.
Regulatory Risks
Fraud incidents kick into action a long list of global compliances and risk mitigation measures. A fraud incident can kick off several investigations and resultant fines, sanctions, and increased scrutiny from regulatory bodies.
For instance, the Australian Securities & Investments Commission (ASIC) recently pursued legal action against HSBC for failing to protect its customers from scams, highlighting the regulatory risks associated with inadequate fraud prevention measures.
Tackling Fraud Globally, One Transaction at a Time
Fraud prevention in the digital world requires a proactive, multi-layered approach. A reactive approach, as we have already seen, is detrimental and even obsolete.
Businesses should double down on real-time fraud detection and AI-driven fraud monitoring and establish constantly evolving security protocols.
Based on the challenges discussed earlier—such as false positives, regulatory risks, and evolving fraud techniques—here’s how you can strengthen your fraud prevention efforts.
Implement AI-driven fraud detection to detect fraud patterns in real time, reducing false positives and improving accuracy.
Strengthen Identity Verification with Multi-Factor Authentication (MFA) like OTPs, biometrics, or security keys to prevent unauthorized account access.
Use Behavioral Biometrics. Tracking user behavior, such as real-time location and device ID, helps detect fraudulent activities.
Deploying Device Fingerprinting prevents fraudsters from using multiple stolen identities on the same device that go unnoticed with the use of Device ID.
Ensure Compliance with Regulatory Standards like PCI DSS, PSD2, and GDPR to ensure secure transactions while minimizing regulatory risks.
Raising user awareness about phishing scams, account takeovers, and fraud prevention best practices can help reduce vulnerability.
How Fraud Analytics Powers Proactive Fraud Detection and Prevention
According to the ACFE’s Occupational Fraud: A Report to the Nations, organizations utilizing proactive data analytics to combat fraud experience a 47% lower fraud loss compared to those without data analytics.
Fraud analytics can help in detecting, preventing, and mitigating fraud with data-driven insights. It helps analyze transaction patterns, user behavior, and historical fraud data, businesses can proactively identify risks and enhance their fraud prevention strategies.
Quantifying Losses and Measuring Fraud Impact
Fraud analytics helps businesses assess the financial impact of fraud. It helps place a finger on the actual cause and effect of fraud while acting as the base to determine the cost of fraud. The same data can also be used to calculate intangible losses that go beyond direct monetary losses.
Identifying Patterns and Fraud Techniques
By analyzing large datasets, fraud analytics detects recurring fraud patterns, common fraud MOs (Modus Operandi), and emerging threats, helping businesses stay ahead of fraudsters.
Pinpointing High-Risk Regions, Devices, and Account Types
Most digital frauds originate from specific regions or are carried out using specific devices. There are also account types that can be associated with certain frauds.
Fraud analytics enables businesses to geo-map fraudulent activities, identify suspicious device fingerprints, and flag risky accounts based on behavioral anomalies.
For example, a digital wallet provider, like Google Pay, detects that a specific city has a surge in account takeovers, prompting targeted security measures for users in that region.
Reducing False Positives and Optimizing Customer Experience
Fraud analytics helps businesses fine-tune fraud detection rules and ensure that their system is not flagging too many false positives. This reduces unnecessary transaction declines and ensures a frictionless customer experience.
Identifying Patterns and Fraud Techniques
The obvious benefit of fraud analytics is that it enables the analysis of large datasets. The analysis can unearth recurring fraud patterns, common fraud MOs (Modus Operandi), and emerging threat models that can help take proactive threat mitigation measures.
Fraud analytics is no longer optional - it's a necessity. By leveraging data-driven insights, businesses can proactively prevent fraud, minimize losses, and strengthen trust with customers.
Frequently Asked Questions (FAQ)
What is fraud detection, and why is it important?
Fraud detection is identifying and preventing fraudulent activities in digital transactions, new account opening, account logins, and operations. Businesses must prevent financial losses, protect customer data, and maintain regulatory compliance.
What are the biggest challenges in fraud detection?
Fraudsters constantly evolve their tactics, making detection difficult.
Balancing fraud prevention with a smooth customer experience.
High false positives can lead to unnecessary transaction declines.
Regulatory compliance requirements add complexity to fraud management.
How should businesses ensure compliance with fraud prevention regulations?
Businesses must follow and keep track of changes around regulations such as PCI DSS (for payment security), PSD2 (for strong customer authentication in Europe), and AML/KYC requirements (for financial institutions). For instance, SAMA's Counter-Fraud Framework mentions the use of authentication measures invloving Behavior and Device for fraud prevention. Fraud analytics helps in generating compliance reports and tracking suspicious activities.

Solutions
Resources
Solutions
Solutions
Industries
Industries
Resources
Resources
Company
Company
Solutions
Solutions
Industries
Industries
Resources
Resources
Company
Company
© 2025 Bureau . All rights reserved. Privacy Policy. Terms of Service.
© 2025 Bureau . All rights reserved.
Privacy Policy. Terms of Service.
Follow Us
Leave behind fragmented tools. Stop fraud rings, cut false declines, and deliver secure digital journeys at scale
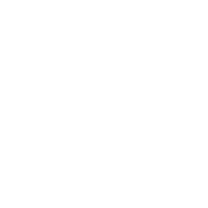
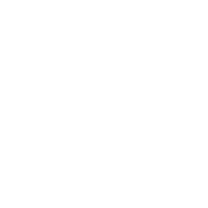

Contact Bureau

Contact Bureau
Leave behind fragmented tools. Stop fraud rings, cut false declines, and deliver secure digital journeys at scale
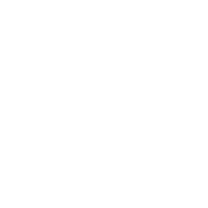
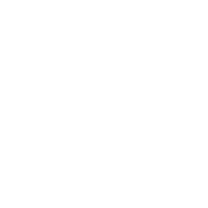

Contact Bureau

Contact Bureau